In today’s digital age, data is the backbone of decision-making across industries, and advertising is no exception. Predicting advertising trends using data is a strategic process that helps businesses stay ahead of the curve, optimize their budgets, and maximize return on investment (ROI). With vast amounts of data generated from social media platforms, web analytics, and consumer interactions, companies have access to invaluable insights that can shape future advertising efforts. But how exactly can data be used to predict advertising trends? Let’s explore the steps involved and best practices for turning data into actionable insights.
Understanding Advertising Trends and Their Importance
Advertising trends are patterns in consumer behavior, technology use, and media consumption that affect how businesses reach their audiences. By anticipating shifts in these trends, advertisers can adjust their strategies proactively, rather than reacting too late. For example, knowing that short-form video content is gaining popularity can guide a business to invest more in platforms like TikTok or Instagram Reels.
Predicting trends allows advertisers to:
- Maximize efficiency: By focusing on the right platforms and formats.
- Enhance targeting: By understanding what resonates with audiences.
- Stay competitive: By adopting new trends before competitors do.
- Optimize budget allocation: By reducing wasteful spending on outdated methods.
Now that we understand why predicting trends is important, let’s dive into the role data plays in this process.
1. Data Collection: Laying the Foundation
The first step in predicting advertising trends is gathering the right data. The quality and scope of your data will directly influence the accuracy of your predictions. Here are the key data sources advertisers can use:
- Web Analytics: Tools like Google Analytics provide valuable insights into user behavior, such as time spent on pages, bounce rates, and conversion paths. This helps identify which content and advertisements engage audiences the most.
- Social Media Data: Platforms like Facebook, Twitter, and Instagram provide insights into engagement metrics (likes, shares, comments), audience demographics, and ad performance.
- Consumer Behavior Data: This includes purchase history, search trends (using tools like Google Trends), and customer reviews. Understanding what consumers are looking for and when can highlight emerging trends.
- Ad Performance Data: Track key performance indicators (KPIs) like click-through rates (CTR), cost-per-click (CPC), and conversion rates. Analyzing which types of ads perform well over time can indicate which formats or messaging strategies are gaining traction.
- Market and Competitor Data: Keep an eye on industry reports, competitor campaigns, and new product launches. Analyzing competitor success and failures can reveal opportunities for improvement in your advertising strategy.
The goal is to accumulate a broad and deep dataset that covers different aspects of the customer journey and market environment.
2. Data Analysis: Uncovering Patterns and Insights
Once the data has been collected, the next step is to analyze it to uncover meaningful patterns. Here are some techniques and tools you can use for data analysis in the context of predicting advertising trends:
a. Trend Analysis
Analyzing historical data can reveal patterns over time. For example, by studying seasonality in sales or consumer behavior, advertisers can anticipate when certain types of ads will be more effective. Seasonal patterns are common in industries like retail and travel. Tools like Google Trends can help identify keywords and topics that are gaining popularity.
b. Audience Segmentation
By segmenting audiences based on demographics, interests, and behaviors, advertisers can identify emerging trends among specific groups. For instance, if younger audiences are increasingly engaging with influencer content, this might indicate a trend worth investing in. Segmentation allows for more personalized advertising, which is known to increase engagement and conversions.
c. Predictive Analytics and Machine Learning
Predictive analytics uses statistical algorithms and machine learning techniques to forecast future outcomes based on historical data. By applying machine learning models to ad performance data, advertisers can predict which types of campaigns are likely to succeed in the future. These models analyze variables like audience behavior, past campaign results, and external factors (e.g., economic conditions) to make informed predictions.
Tools like Google Analytics 4, HubSpot, and more advanced platforms like IBM Watson and Tableau can be used to build predictive models. The more sophisticated the model, the better it can forecast future trends by accounting for various factors and interactions.
d. Sentiment Analysis
Sentiment analysis involves using natural language processing (NLP) to gauge the emotions and opinions expressed in online content such as social media posts, reviews, and forums. Advertisers can use this technique to measure how consumers feel about specific brands, products, or trends. For example, if there is a surge in positive sentiment around eco-friendly products, it could indicate a growing trend toward sustainability in advertising.
3. Identifying and Acting on Emerging Trends
With data analysis complete, advertisers can start identifying trends and making predictions. However, recognizing an emerging trend is only part of the equation. The real value comes from acting on this knowledge. Here’s how advertisers can turn insights into action:
a. Content Creation Aligned with Trends
Based on trend predictions, advertisers can create content that resonates with their audience’s current interests. For instance, if data shows that interactive ads (e.g., polls or quizzes) are gaining engagement, brands can incorporate these elements into their campaigns.
b. Platform and Format Optimization
Data might reveal that a certain platform is becoming more popular with a specific demographic, or that a particular ad format (e.g., video ads, carousel ads) performs better. Advertisers should shift their focus and budget toward these platforms and formats to maximize engagement and conversions.
For example, if data suggests that Gen Z is increasingly consuming video content on TikTok, brands targeting this demographic should prioritize video content on that platform.
c. Budget Allocation Based on Predictive Insights
Predictive models can help advertisers allocate their budgets more effectively by forecasting which campaigns are likely to deliver the best ROI. For instance, if historical data shows that pay-per-click (PPC) campaigns generate higher conversion rates during specific times of the year, advertisers can adjust their budgets accordingly. If you want to find great tips and ideas about how to use data to predict advertising trends, click here to find out more information.
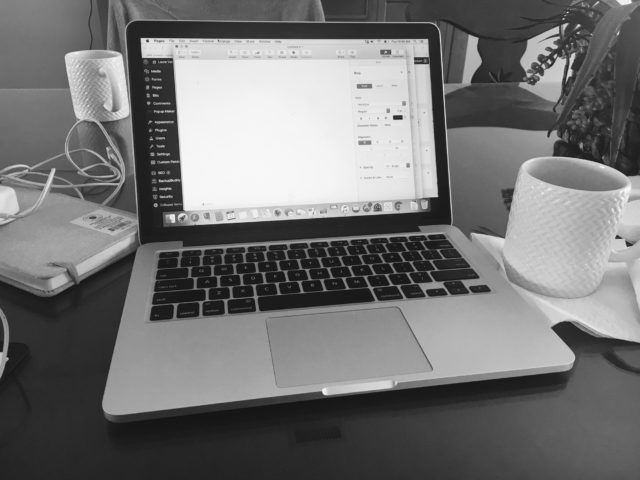
4. Continuous Monitoring and Adaptation
The advertising landscape is always evolving, and trends can shift rapidly. This is why continuous monitoring of data is crucial. Advertisers should regularly revisit their predictive models and trend analysis to ensure they remain relevant and up to date.
a. A/B Testing
A/B testing, or split testing, allows advertisers to test different versions of an ad to determine which performs better. This is a form of real-time data collection that provides insight into audience preferences. By continuously testing and optimizing, advertisers can stay aligned with current trends and improve ad performance over time.
b. Real-Time Analytics
Real-time data tools provide immediate insights into how ads are performing across different platforms. This enables advertisers to make quick adjustments, such as reallocating budget to higher-performing campaigns or tweaking ad creatives based on real-time feedback.
Conclusion
Using data to predict advertising trends is not only about staying relevant but also about creating more effective, personalized, and impactful campaigns. By collecting the right data, applying advanced analytics, and staying agile in response to emerging trends, advertisers can position themselves for long-term success. The future of advertising will be increasingly driven by data, artificial intelligence, and machine learning. Brands that embrace these technologies and refine their data-driven strategies will be the ones leading the way in a rapidly changing digital landscape.
Data-driven insights empower advertisers to predict what will resonate with their audience next and to act accordingly. The key is to remain proactive and adaptive, continuously leveraging the power of data to stay ahead of the competition.